
We finally compared expected and observed shrinkage as well as the predicted and estimated Standard Errors (SE) of individual parameters. We then evaluated the approximation of M BF FO and compared it to Monte-Carlo (MC) simulations. Shrinkage values were generated for parameters of two pharmacokinetic models by varying the structure and the magnitude of the random effect and residual error models as well as the design. From the shrinkage formula in linear mixed effects models, we derived the predicted shrinkage from M BF. We 1) Propose and evaluate an approximation of M BF by First-Order linearization (FO), 2) Explore by simulations the relationship between shrinkage and precision of estimates and 3) Evaluate prediction of shrinkage and individual parameter precision. The objective of this work was to predict individual parameter shrinkage from the Bayesian information matrix ( M BF).
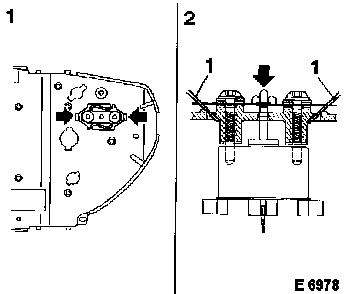
When information is sparse, individual parameters derived from a non-linear mixed effects model analysis can shrink to the mean.
